Speaker
|
Biography
|
Abstract
|
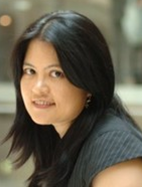
Min-Hua Jen
(Eli Lilly)
|
Min-Hua Jen is currently Senior Director - Real-World & Access analytics at Eli Lilly, leading the International Business team on Market Access/HEOR/Medical affairs statistical support. She has extensive experience applying statistics to clinical research, epidemiology and health economics and outcomes research in academia and industry settings. She is an active member in the PSI/EFSPI HTA Special Interest Group (SIG) and the engagement chair of the ISPOR Oncology SIG. She was trained in Epidemiology and Statistics and obtained her PhD at University of Bristol. Her research interests including indirect treatment comparisons and network meta-analysis; particularly incorporate external data for time to event outcomes, surrogacy analyses, multilevel modelling and health economic modelling.
|
The Regulation on health technology assessment (HTAR) will apply from January 2025. It will not only affect all statisticians in the pharmaceutical industry with respect to skill sets and collaboration, but will also have impact on statistical approaches used to obtain the effect estimates. The estimates would be needed for the economic model which impact on the reimbursement and pricing negotiation subsequently in many European countries.
Before diving deeper into the subjects of the topic for each webinar, Min-Hua as the event moderator will provide a short introduction of the HTAR, the outline of each webinar, and the speakers. She will then lead the panel discussions.
|
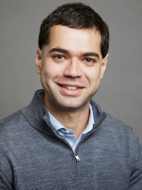
Antonio Remiro-Azocar
(Bayer)
|
Antonio is Lead Medical Affairs Statistician at Bayer, specializing in health technology assessment (HTA), health economics and outcomes research (HEOR), and observational science. At Bayer, Antonio leads cross-functional teams in providing inputs to life-cycle management strategies, publication plans, reimbursement requirements, HTA studies and analyses for payers, across a number of therapeutic areas. Prior to his current role, Antonio provided support on the statistical aspects of evidence synthesis, HTA and HEOR to contract research organizations such as IQVIA and ICON plc, and public bodies such as SickKids. Antonio holds a PhD in Statistical Science and an MSc in Machine Learning from University College London.
|
Marginal versus conditional estimands, non-collapsibility and standardization in the context of indirect treatment comparisons
There is active debate among researchers about whether marginal or conditional treatment effect estimands are more appropriate inferential targets for health technology assessment. Marginal estimands are widely regarded as more appropriate for population-level decision making. In indirect treatment comparisons, challenges arise from the conflation and potential incompatibility of marginal and conditional estimands. This is particularly the case when effect measures are non-collapsible. Model-based marginalization or standardization methods allow one to produce marginal, but covariate-adjusted, treatment effect estimates that are compatible in indirect treatment comparisons.
Established covariate-adjusted indirect comparison approaches, e.g., matching-adjustment, can be viewed as methods that marginalize over the covariate distribution of an external study. Currently available covariate adjustment methods for indirect treatment comparisons will be outlined, and their advantages and disadvantages will be highlighted. The implications of non-collapsibility to currently recommended practices in evidence synthesis, the assessment of effect measure modification and heterogeneity, and the definition of estimands with limited access to subject-level data, will be discussed. Standard practices may require updating in light of conflicting views and the issues discussed.
|
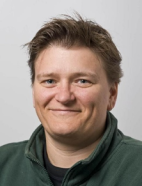
Anja Schiel
(Norwegian Medical Products Agency (NoMA))
|
Anja Schiel has studied Biology at the Johannes Gutenberg-University, Mainz, Germany. She received her PhD from the Free University in Amsterdam in 2006 and worked several years as Post-Doc on a range of subjects focusing on oncology, immunology and molecular biology, first at the University of Leiden and later at the University of Oslo, before starting at the Norwegian Medicines Agency (NoMA) in 2012.
At NoMA she is working as Special Adviser/Statistician/Methodologist both on regulatory (EMA) and HTA projects. She has been Chair of EMA’s Biostatistics Working Party (2017 – 2019) and during 2019 – 2022 she was Chair of the Scientific Advice Working Party (SAWP) at EMA. She continues currently as alternate member at the SAWP and is member of the new Methodology Working Party (MWP) recently established at EMA.
She has been involved in EUnetHTA JA3 and its successor, EUnetHTA 21, with particular focus on parallel EMA-HTA scientific advice (joined scientific consultations). As one of the vice Chairs of the JSC Committee for Scientific Consistency and Quality (JSC CSCQ) she was involved in the preparation of the implementation of developer support under the Regulation on Health Technology Assessment (HTAR, to apply from January 12th 2025). Currently she is a member of the Joined Scientific consultation sub-group (JSC SG) and alternate Member in the Methodological and Procedural sub-group (MPG SG).
|
ITC’s applicability and acceptability in the European HTA landscape
In 2025 the Regulation on health technology assessment (HTAR) will become operational and all involved Stakeholders are in preparation for this major change in the EU reimbursement landscape.
Among the questions remaining is one on how the EU members states will handle their methodological differences when it comes to health technology assessment at the national level.
Today we will look at the topic of indirect treatment comparisons and what is currently known based on the existing guideline drafted during the EUnetHTA21 tender period and ongoing work in the Methodological and Procedural Guidance sub-group (MPG) of the HTAR coordination group (HTAR CG).
|
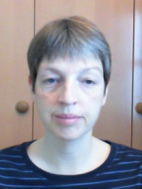
Suzy Van Sanden
(Johnson & Johnson)
|
Suzy first studied mathematics, followed by a Master of Science in Biostatistics. In 2008 she obtained my Ph.D in Biostatistics at Hasselt University, where she also taught classes in mathematics and statistics. Afterwards, she continued her research during a 2-year postdoc, in close collaboration with Janssen. She then moved to the market access department in Janssen where she still works as a lead statistician and methods expert to support the many reimbursement dossiers in the EMEA region.
|
Matching-adjusted indirect comparison (MAIC): a case studies in prostate cancer
Apalutamide and enzalutamide are next-generation androgen receptor inhibitors that both demonstrated efficacy in separate placebo-controlled trials when used in combination with androgen deprivation therapy (ADT) for the treatment of non-metastatic castration-resistant prostate cancer (nmCRPC). In the absence of comparative trials between these agents, the present study sought to indirectly compare metastasis-free survival (MFS) and overall survival (OS) in patients with nmCRPC who received these therapies.
An anchored matching-adjusted indirect comparison (MAIC) was conducted by weighting individual patient-level apalutamide data to match the baseline characteristics of published aggregate enzalutamide data. Hazard ratios (HRs) for MFS and OS were re-estimated using weighted Cox proportional hazards models and indirectly compared using a Bayesian network meta-analysis.
MAIC based HRs were favourable for apalutamide versus enzalutamide for both MFS and OS. The Bayesian probabilities of apalutamide being more effective than enzalutamide were 73.6% for MFS and 83.5% for OS. These results suggest that nmCRPC patients treated with apalutamide have a higher probability of a more favourable MFS and OS compared with those treated with enzalutamide.
|
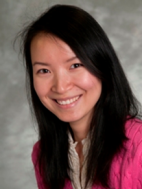
Kate Ren
(University of Sheffield)
|
Kate Ren is a Senior Research Fellow in statistics at the University of Sheffield. She works as a member of SCHARR-TAG (an external assessment group for NICE technology appraisals). She has extensive experience in critiquing evidence synthesis methods used in NICE single technology appraisal submissions. She is also a member of NICE Appraisal Committee C. Her research interests include indirect treatment comparisons, survival extrapolation and structured expert elicitation.
|
Unanchored indirect treatment comparison methods and unmeasured confounding
This presentation will introduce two unanchored indirect treatment comparison methods: matching-adjusted indirect comparison (MAIC) and simulated treatment comparison (STC). These methods are used for estimating treatment effects from single-arm studies in health technology assessment where individual patient-level data (IPD) are available for one treatment only; for the comparator treatment only published summary statistics are available. This scenario commonly arises when a pharmaceutical company only has IPD for its own intervention.
Both unanchored MAIC and STC rely on the strong assumption that all prognostic factors and effect modifiers are accounted for and there is no residual confounding. This assumption is usually impossible to meet in practice because the selection of the covariates is constrained by what baseline characteristics are reported in the comparator trial.
A quantitative bias analysis approach for unmeasured confounders will be introduced to address a limitation of MAIC and STC when certain prognostic factors and/or effect modifiers are not reported in the comparator trial. I will demonstrate how this approach provides a quantitative assessment of the impact of the bias associated with unmeasured confounders.
|
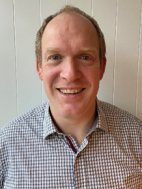
Andrew Thomson
(EMA)
|
Andrew Thomson is a statistician with over 16 years experience in the regulatory system. He is currently at the EMA, in the Taskforce dedicated to Data, Analytics and Methodology. Here his tasks include being part of the Scientific Secretariat for the Methodology Working Party, as well as the Regulatory Chair for ICH E11A (Paediatric Extrapolation) and ICH E6 Annex 2 (GCP for trials incorporating decentralised and pragmatic elements and using Real World Data). Prior to joining the EMA in 2014, he spent 7 years at the UK Regulator, the MHRA, initially as a Statistical Assessor in the Licensing Division, and subsequently Head of Epidemiology in the Vigilance & Risk Management of Medicines Division.
|
The role of single-arm trials in the authorisation of new medicines
In this session, Marcia and Andrew will present on the regulatory acceptance of single-arm trials. This will focus on their historic uses, how decision making is made based on them, and how different regulatory decisions may make use of the information, including the utility and need for indirect comparisons. The recently published "Single-arm trials as pivotal evidence for the authorisation of medicines in the EU” will be discussed. This covers topics including the choice of endpoint, the trial population, the use of external information, and a discussion on sources of bias and how to address them.
|
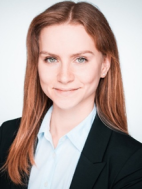
Marcia Rückbeil
(EMA)
|
Marcia has a master’s degree in Mathematics and a Ph.D. in Biostatistics. She joined the Data Analytics and Methods Task Force at the European Medicines Agency as a Seconded National Expert in 2020. In this role, she contributed to EMA’s reflection paper on single-arm trials, which was subject to public consultation in 2023. Before joining the European Medicines Agency, she worked in academia for five years.
|
The role of single-arm trials in the authorisation of new medicines
In this session, Marcia and Andrew will present on the regulatory acceptance of single-arm trials. This will focus on their historic uses, how decision making is made based on them, and how different regulatory decisions may make use of the information, including the utility and need for indirect comparisons. The recently published "Single-arm trials as pivotal evidence for the authorisation of medicines in the EU” will be discussed. This covers topics including the choice of endpoint, the trial population, the use of external information, and a discussion on sources of bias and how to address them.
|
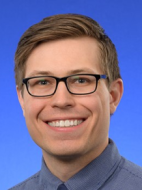
Richard Sizelove
(Eli Lilly)
|
Richard Sizelove is a Research Advisor – Statistics, Oncology at Eli Lilly & Company. Richard joined Lilly after receiving his PhD in Biostatistics from the University of North Carolina at Chapel Hill. His research interests include interval-censored survival analysis, semiparametric inference, and indirect treatment comparisons.
|
Performance of MAIC for single-arm trials: a simulation study
Matching adjusted indirect comparison (MAIC) has become an increasingly popular method to conduct population adjustment for indirect comparisons between single-arm studies. The assumptions required for performing MAIC in this setting are strict, so there is need to empirically assess the method’s operating characteristics. Previous simulation studies evaluating MAIC for single-arm trials ignore the non-collapsibility of the treatment effect measure and therefore incorrectly specify the target of estimation. We remedy this error and conduct a comprehensive simulation study to assess the performance of MAIC for single-arm trials in a variety of scenarios.
|