Speaker
|
Biography
|
Abstract
|
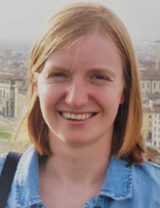
Kelly van Lancker
|
Dr. Kelly Van Lancker is a postdoctoral researcher in biostatistics at Ghent University, Belgium, where she also obtained her PhD. Previously, Kelly was a postdoctoral researcher at the Johns Hopkins Bloomberg School of Public Health. Her goal is to develop innovative designs and analytical techniques for drawing causal inferences in health sciences. A big part of her research focuses on more accurate and faster decision-making in randomized clinical trials by making optimal use of the available data. She thereby mainly focuses on covariate adjustment, data-adaptive methods, complex designs, estimands and especially a combination of these topics. Her recent research is primarily aimed at learning about the opportunities and challenges in running pragmatic trials within clinical practice, and developing better prediction tools for personalized medicine.
|
The role of causal inference in clinical trials: an introduction
In light of the recently published ICH E9(R1) guideline on estimands and sensitivity analysis (2019) and the FDA draft guideline on covariate adjustment (2023), causal inference is progressively taking a more prominent role in the landscape of global drug development. In this talk we will try to bridge the gap between communities by elaborating on how this field provides a convenient, unifying framework, language and relevant tools to formally establish causal relationships. We will hereby illustrate how causal thinking, combined with important tools such as potential outcomes, can facilitate defining, identifying and estimating treatment effects. Building on this, we discuss the role of causal inference in different trial settings, including targeting intention-to-treat effects with covariate adjustment, handling intercurrent events and the incorporation of external control data.
|
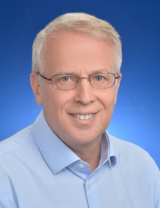
Ilya Lipkovich
|
Ilya Lipkovich is a Sr. Research Advisor at Eli Lilly and Company. Ilya received his Ph.D. in Statistics from Virginia Tech in 2002 and has > 20 years of statistical consulting experience in pharmaceutical industry. He is an ASA Fellow and published on subgroup identification in clinical data, analysis with missing data, and causal inference.
|
Causal inference and estimands in clinical trials
This presentation revisits recent ICH E9 (R1) Addendum on Estimands and Sensitivity Analysis in Clinical Trials and discusses various strategies for handling intercurrent events (ICEs) using the causal inference framework. The language of potential outcomes (PO) is widely accepted in the causal inference literature but is not yet recognized in the clinical trial community and was not used in defining causal estimands in ICH E9(R1). I will try to bridge the gap between the causal inference community and clinical trialists by advancing the use of causal estimands in clinical trial settings and illustrate how concepts from causal literature, such as POs and dynamic treatment regimens, can facilitate defining and implementing causal estimands for different types of outcomes providing a unifying language for both observational and randomized clinical trials. I emphasize the need for a mix of strategies in handling different types of ICEs, rather than taking one-strategy-fit-all approach and suggest that hypothetical strategies should be used more broadly and provide examples of different hypothetical strategies for different types of ICEs.
|
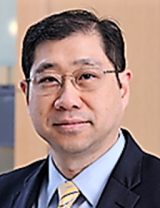
Martin Ho
|
Martin Ho is an ASA Fellow, and a Senior Director of RWE Rare Disease, Evidence Generation Platform at Pfizer, leading all RWE activities for Sickle Cell Disease assets. Prior, he was the head of biostatistics at Google, LLC for 2 years and served the public at the U.S. FDA for 13 years, with the last 3 years as Associate Director of the Office of Biostatistics and Epidemiology at the Center for Biologics Evaluation and Research. Before joining the U.S. FDA, he worked as senior biostatisticians in contract research organizations for clinical studies. He co-led the RWE Scientific Working Group of the ASA Biopharmaceutical Section and the Working Group published 5 papers in landscape assessments as well as state of the science prospects of RWE for regulatory considerations. One of the papers introduces a casual inference roadmap for RWE design and analysis in regulatory context.
|
A Causal Inference Roadmap for Generating RWE in Regulatory Context: An Introduction and Illustration
As real-world data (RWD) become more readily available, the regulatory agencies, medical product developers, and other key stakeholders has increasing interests in exploring the use of real-world evidence (RWE) to support regulatory decisions alternative to traditional clinical trials. To facilitate and promote statistical research in design, analysis, and interpretation of RWE studies for regulatory decision making, the ASA Biopharmaceutical Section established the RWE Scientific Working Group to address challenges and identify opportunities in the statistical research of this area. In a Working Group publication in 2022, Ho and colleagues (DOI 10.1080/19466315.2021.1883475) have proposed a causal inference roadmap for study design and analysis that generates RWE for regulatory considerations. In this talk, Martin will briefly review the steps of the roadmap before using an example to illustrate how to apply the roadmap to generate RWE for regulatory consideration.
|
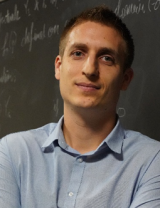
Alex Ocampo
|
I am currently a statistician with Novartis based in Basel, Switzerland. I obtained my Bachelor’s degree in Statistics from the University of Michigan in my hometown of Ann Arbor and then a Ph.D. in Biostatistics from Harvard University in 2020. My doctoral dissertation focused on statistical methods for dealing with missing data when the “Missing at Random” assumption does not hold. My current work at Novartis focuses on leveraging causal reasoning in the pharmaceutical industry.
|
Single-World Intervention Graphs for Defining, Identifying, and Communicating Estimands in Clinical Trials
Confusion often arises when attempting to articulate target estimand(s) of a clinical trial in plain language. We aim to rectify this confusion by using a type of causal graph called the Single-World Intervention Graph (SWIG) to provide a visual representation of the estimand that can be effectively communicated to interdisciplinary stakeholders. These graphs not only display estimands, but also illustrate the assumptions under which a causal estimand is identifiable by presenting the graphical relationships between the treatment, intercurrent events, and clinical outcomes. To demonstrate its usefulness in pharmaceutical research, we present examples of SWIGs for various intercurrent event strategies specified in the ICH E9(R1) addendum, as well as an example from a real-world clinical trial for chronic pain. LaTeX code to generate all the SWIGs shown is this paper is made available. We advocate clinical trialists adopt the use of SWIGs in their estimand discussions during the planning stages of their studies.
|
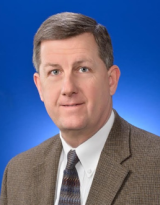
Stephen Ruberg
|
Stephen Ruberg, PhD spent 38 years in the pharmaceutical industry and is currently the founder and President of Analytix Thinking, a consulting company dedicated to advancing the use of statistics in the design of clinical trials, the conduct of analysis, and the interpretation of scientific data. His present interests are in estimands, subgroup identification, Bayesian statistics and digital medicine. He is a Fellow of the American Statistical Association, the International Statistics Institute and was a member of an advisory committee to the Secretary of Health and Human Services in the US federal government.
|
Estimating Treatment Effects in Patients Who Adhere to Treatment (Part 1)
The estimation of treatment effects has traditionally been based on the value of randomization and the causal inference it confers. However, causal inference from randomized controlled trials requires that all patients be analyzed as randomized AND, importantly, that all patients be followed for the duration of the trial and the primary outcome measured. Since many large or long-term trials involve patients who discontinue the study or discontinue their study treatment, this approach – often called intent-to-treat (ITT) – actually becomes an estimate of the effect of initiating (or being assigned) a treatment and NOT the effect of actually taking the treatment, which we call the direct treatment effect. An alternative approach is to censor the data from the time of treatment deviation and impute the resulting missing values (e.g., a hypothetical strategy). This approach uses all randomized patients but requires strong assumption on the potential outcome after the deviation away from the randomized treatment. While ICH-E9 recommended the ITT approach in general (or at least the use of all randomized patients in the analysis), ICH-E9(R1) has opened the door to other possible estimands and strategies for estimating a treatment effect. One such alternative is the direct treatment effect in patients (principal stratum) who actually would take/adhere to a treatment (Adherers Average Causal Effect – AdACE). This lecture will be divided into two parts: the first will motivate why such an estimand is of major importance, and the second will provide technical details on its estimation using causal inference methods. Examples will be given to highlight the methods, the code needed, and the interpretation of such the AdACE estimate.
|
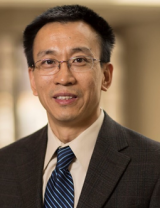
Yongming Qu
|
Yongming Qu is currently a Vice President at Eli Lilly and Company. He received his PhD in Statistics from Iowa State University in 2002. He has made significant contributions in all phases of clinical development at Lilly and has been active in research for using novel analytics and statistical methods in drug development. He is a Fellow of American Statistical Association.
|
Estimating Treatment Effects in Patients Who Adhere to Treatment (Part 2)
(Abstract as above)
|
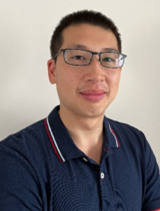
Sean Yiu
|
Sean is a Principal Statistical Scientist at Roche, UK. Prior to this, he was a Research Associate at the MRC Biostatistics Unit, University of Cambridge, which is also where he obtained his PhD in biostatistics. At Roche, Sean works on establishing new endpoints in neuroscience, understanding the operating characteristics of treatments on clinical outcomes through characterizing drug concentration-response relationships and disentangling distinct pathways from treatment to outcomes via potential mediators, as well as on real world observational studies for making indirect treatment comparisons and estimating long-term treatment effects. He also supports the conduct of ongoing Phase 3 and post marketing requirement studies in multiple sclerosis as a study statistician, and collaborates with academics, external companies and multiple sclerosis registries in his projects. His research interests include longitudinal data, causal inference, missing data and composite endpoints.
|
Comparative safety analysis of time-varying exposures in post marketing observational studies
Health authorities often mandate license holders of approved treatments to conduct post marketing observational studies to sufficiently assess long-term risk of important safety events, e.g. malignancies, since randomized clinical trials are typically too short and underpowered to detect treatment effects on such events. Furthermore, comparative safety analysis of newly approved versus other already approved treatments may be requested as part of the post marketing requirement. However, performing comparative safety analysis of long-term observational studies where treatment assignment is based on clinical practice is challenging and not well established in the regulatory setting, particularly when treatment switching (from control to active and vice versa) is anticipated to be frequent and often occurs prior to safety events of interest. Using a case study for OCREVUS, which is an approved treatment for adult patients with relapsing or primary progressive forms of multiple sclerosis, I will describe one specific post marketing requirement from the FDA on comparative safety analysis, the challenges of performing such analyses in the presence of multiple treatment switching, and highlight severe limitations of conventional methods based on time fixed treatments. I will then describe how established methodology for drawing causal inferences for the effects of time-varying exposures in the presence of time-dependent confounding, e.g. marginal structural Cox models, can address limitations of the conventional methods, and provide feedback from the FDA on the use of causal inference methodology in this observational setting.
|
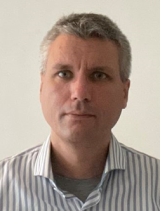
Martin Linder
|
Martin is a statistician with close to 15 years experience in the pharmaceutical industry and currently employed by Novo Nordisk A/S. He combines regular activities in clinical trials with an interest in statistical methodology, including the use of causal inference.
|
Mediation analysis for a cardiovascular outcome trial
There is a growing interest in statistical analyses that can answer questions concerning how a drug may affect an outcome via intermediate variables (mediators). The LEADER trial is an example. The trial showed a beneficial effect of the drug liraglutide on cardiovascular outcome in people with type 2 diabetes and high cardiovascular risk. Key opinion leaders as well as regulatory agencies asked whether the effect on cardiovascular outcome could be explained by previously known effects of liraglutide on blood glucose levels or body weight. The question is best answered within the framework of causal inference which provides methods for statistical analysis but also clarifies the assumptions necessary for a meaningful interpretation of the results.
In this presentation, we will consider some selected methods for causal mediation analysis that will be applied to the LEADER data. The methods include an approach developed jointly with experts from academia which specifically handles the case where the outcome is a time-to-event variable and the mediator is repeatedly measured.
|