PSI One Day Meeting: New Emerging Topics around Estimands and ICH Addendum
IQVIA, 500 Brook Drive, Reading, RG2 6UU, UK
The draft ICH E9 addendum on estimands and sensitivity analysis was released back in July 2017 and (more than 1000) comments are back. All stakeholders are gaining the necessary experience and familiarity with estimands along with the associated challenges and methodologies. The language and thinking behind causal inference is well suited to this area.
The PSI Scientific Committee have put together this one day meeting to share and discuss new emerging topics around estimands and the ICH addendum. The aims of the event are to:
- Share the feedback from the public consultation on the draft ICH E9 addendum
- Explore the estimand concept within health technology assessments
- Describe how casual inference fits into the area of estimands
- Present case studies illustrating the implementation of the estimand framework and the use of causal inference methodology
Agenda
09:30 - 10:00 |
Registration, Welcome and introduction
|
10:00 - 10:40 |
ICH E9 addendum: Key themes raised during public consultation
Chrissie Fletcher (Amgen on behalf of the ICH E9 Working Group)
|
10:40 - 11:20 |
The exciting new world of the ‘Estimand’
Anja Schiel (Norwegian Medicines Agency)
|
11:35 - 12:15 |
Estimand and analysis considerations of Phase 3 clinical trials involving CAR-T – A case study in lymphoma
Evgeny Degtyarev, on behalf of Novartis team
|
12:15 - 12:55 |
How causal inference can fit the needs of a clinical trial (well kind of)
Michael O’Kelly (IQVIA)
|
13:45 - 14:25 |
Using causal graphs to understand estimands and estimation
Ian White (UCL)
|
14:25 - 15:05 |
Towards more reliable Mendelian randomization investigations
Stephen Burgess (University of Cambridge)
|
15:25 - 16:05 |
Non-inferiority case study
Oliver Keene (GSK)
|
16:05 - 16:30 |
Panel Discussion
Speakers and Yolanda Barbachano (MHRA)
|
Abstracts
Chrissie Fletcher
(Amgen on behalf of the ICH E9 Working Group)
|
ICH E9 addendum: Key themes raised during public consultation
Abstract: The draft ICH E9(R1) and addendum to E9 incorporating a new framework on estimands and sensitivity analyses in clinical trials was released for public consultation in the first region at the end of August 2017 and the public consultation ended in the last region by the end of April 2018. The ICH E9 working group met in June 2018 to review the 1200+ comments that were submitted. The ICH E9 addendum and E9(R1) is scheduled to reach step 4 and sign-off by all the ICH regions in June 2019.
The key themes and topics raised during the public consultation of the ICH E9 addendum will be presented. A summary of the E9 working group discussion of the key aspects raised during public consultation and an update of how the E9 working group are trying to address the comments in the final E9 addendum will be provided. |
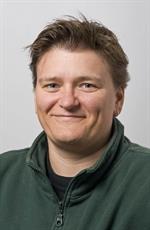
Anja Schiel (Norwegian Medicines Agency)
|
The exciting new world of the ‘Estimand’
Abstract: In August 2017 the ‘ICH E9 (R1) Addendum on estimands and sensitivity analysis in clinical trials to the guideline on statistical principles for clinical trials’ was released for consultation and has stirred discussion and excitement, mainly in the regulatory world. But, what does this new framework offer to other stakeholders? The uptake of new concepts into health technology assessment is notoriously difficult because there are fundamental differences in the evidence requirements needed to establish a risk-benefit and those to establish relative effectiveness. The Estimand framework offers new possibilities to allow studies to be designed with both perspectives in mind, wherever possible. To designs studies based on the Estimand concept requires a wider communication between the different specialists involved in drug development, from pharmacologists to clinical experts and statisticians. However, this dialogue should in fact be expanded all the way to the health economists to fully harness the potential of this new framework.
|
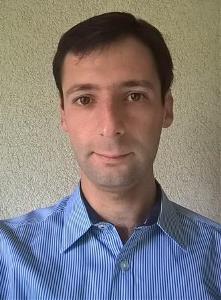
Evgeny Degtyarev, on behalf of Novartis team |
Estimand and analysis considerations of Phase 3 clinical trials involving CAR-T – A case study in lymphoma
Abstract: Marked by the recent approval of the first chimeric antigen receptor T cell (CAR-T) therapies, these autologous therapies provided patients with new options to fight cancer. Unique challenges arises in the design and analysis of randomized studies involving autologous CAR-T therapies. Because the CAR-T treatment strategy involves personalized manufacturing before patients can receive the final product, the scientific objective and its associated estimand must be carefully thought through to allow appropriate interpretation of study results. Different testing procedures and estimation methods will be discussed in a case study of Phase 3 clinical trial.
|
Michael O’Kelly (IQVIA)
|
How causal inference can fit the needs of a clinical trial (well kind of)
Abstract: Randomisation can be thought of as providing “a ‘reasoned basis’ of testing the null hypothesis of no effect without resort to distributional assumptions such as normality” (Fisher); and indeed randomisation has been accepted as providing as close to causal inference as was needed for the approval of new treatments. However, clinical triallists are now seeing that, over the time of follow-up, intercurrent events (ICEs) result in changes to treatment. Because of this, the planned trial of a randomized treatment regimen can morph into no more than a survey whose only inference from randomisation is confined to the mere act of assigning a plan of treatment, a survey whose inference about the treatment regimen itself loses much of its credibility because those ICEs constitute non-randomized changes and distortions of the regimen to be tested. This presentation tries to convey in a non-technical manner the idea of causal inference and how it can work and be of use in clinical trials, making at least a gesture towards inference about outcomes as actually planned. Noting the overlap with missing data research, the presentation then shows a detailed example of the use of one approach to causal inference for an outcome censored by death. From the example it may be concluded that, while causal inference is probably invaluable for many clinical trial designs including the example presented, results from causal inference have their own limitations and will often need to be interpreted alongside other results, even if the other results are more open to bias than those from causal inference.
|
Ian White (UCL)
|
Using causal graphs to understand estimands and estimation
Abstract: I will talk about causal inference and estimands from the perspective of DAGs (directed acyclic graphs), which are widely used for causal inference in observational studies. DAGs allow complex statistical issues to be represented pictorially yet still rigorously. I will briefly describe the ideas of DAGs, then draw suitable DAGs for randomised trials with non-randomised treatment changes, and use them to discuss some key estimands and how these may be estimated. The talk will be conceptual rather than mathematical, and will point to types of approach rather than specific approaches.
|
Stephen Burgess (University of Cambridge)
|
Towards more reliable Mendelian randomization investigations
Abstract: Mendelian randomization is a technique for assessing the causal role of a modifiable risk factor on a disease outcome using genetic data. If genetic variants associated with the risk factor are also associated with the outcome, this increases the plausibility that the risk factor is a causal determinant of the outcome. However, if the genetic variants in the analysis do not have a specific biological link to the risk factor, then causal claims can be spurious. Recent advances in genome-wide association studies and the increasing availability of publicly available summary data on associations of genetic variants with risk factors and disease outcomes in large sample sizes have enabled powerful Mendelian randomization analyses to be performed relatively quickly and simply. I will present an overview of Mendelian randomization approaches from monogenic analyses, in which genetic variants are taken from a single gene region, to polygenic analyses, which include variants from multiple regions. In particular, I will discuss the reliability of such analyses and present statistical approaches for increasing their reliability.
|
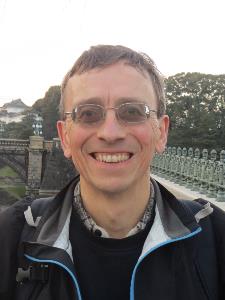
Oliver Keene (GSK) |
Non-inferiority trials and the ICH E9 estimands framework
Abstract: The estimand framework described in the draft addendum to the ICH E9 regulatory guideline has led to a reappraisal of non-inferiority trials. Currently, these trials often make use of a Per Protocol Population where subjects who violate the protocol are excluded. In the estimands framework, protocol violations after randomisation can be seen as intercurrent events and subjects who do not fulfil inclusion/exclusion criteria can potentially be excluded based on the population of interest. Therefore, the role of a Per Protocol Population is substantially diminished or eliminated entirely.
The strategy to be employed for intercurrent events such as discontinuation of randomised treatment in the non-inferiority setting has not been established yet. For example, some statisticians favour use of a hypothetical strategy for key intercurrent events, while others believe a treatment policy strategy provides more robust estimation.
This talk will use a case study of a trial in COPD to illustrate how to implement the estimands framework to a non-inferiority trial. The talk will discuss the application of different strategies for intercurrent events in the non-inferiority setting and what additional comparisons of intercurrent events will be helpful.
|
PSI Member |
£40 + VAT |
Non-Member |
£135 + VAT (This includes PSI membership for 2019) |
Registration is now closed.
If you require overnight accommodation before or after the event the closest hotels are
Millennium Hotel and
Hilton. Reading is a large town with numerous accommodation options in the town centre a short distance away. There is a
Premier Inn amongst others. Delegates should book directly with hotels.